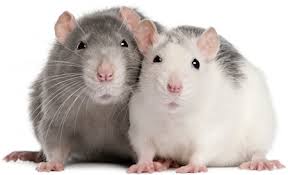
Artificial intelligence in forecasting future infectious disease outbreaks
Machine learning can pinpoint rodent species that harbor diseases and geographic hotspots vulnerable to new parasites and pathogens. So reports a new study in the Proceedings of the National Academy of Sciences led by Barbara A. Han, a disease ecologist at the Cary Institute of Ecosystem Studies.
Most emerging infectious diseases are transmitted from animals to humans, with more than a billion people suffering annually. Safeguarding public health requires effective surveillance tools.
With University of Georgia Odum School of Ecology colleagues John Paul Schmidt, Sarah E. Bowden, and John M. Drake, Han employed machine learning, a form of artificial intelligence, to reveal patterns in an extensive set of data on more than 2,000 rodent species, with variables describing species’ life history, ecology, behavior, physiology, and geographic distribution.
A Cary Institute release reports that the team developed a model that was able to predict known rodent reservoir species with 90 percent accuracy, and identified particular traits that distinguish reservoirs from non-reservoirs. They revealed over 150 new potential rodent reservoir species and more than fifty new hyper-reservoirs (animals that may carry multiple pathogens infectious to humans).
By combining ecological and biomedical data into a common database, Barbara was able to use machine learning to find patterns that can inform an early warning system for rodent-borne disease outbreaks.
The riskiest reservoir species are those that mature quickly, reproduce early and often, and live northern temperate areas with low levels of biodiversity.
Geographic areas found to have a high diversity of rodent reservoirs included North America, the Atlantic coast of South America, Europe, Russia, and parts of Central and East Asia. Predicted future hotspots of rodent reservoir diversity spanned arctic, temperate, tropical, and desert biomes, including China, Kazakhstan, and the Midwestern United States. A majority of new reservoir and hyper-reservoir species are predicted to occur in the upper latitudes.
Findings provide a basis for targeted surveillance efforts, which are vital given the cost of monitoring for emerging infectious diseases.
The release notes that the machine learning techniques applied in this study are currently being used to explore new questions, including potential reservoirs of Ebola virus and other filoviruses.
Full article here.